LNOB assessment in Nepal: Data landscaping in Tulsipur municipality
This report, co-produced with BASE, draws on DI's data landscaping work in Tulsipur, Nepal. It explains how to improve the data ecosystem to leave no one behind.
Downloads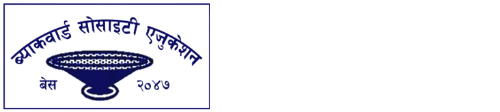
Our work on data landscaping in Nepal was carried out in collaboration with Backward Society Education (BASE) who gathered the data presented in this report.
Introduction
Leave no one behind (LNOB) is the central transformative promise of the 2030 agenda. It compels development actors to consider the furthest behind first and to tackle the discrimination and exclusion that drive inequality. Within Development Initiatives’ (DI’s) Poverty and Inequality (P&I) programme, we use our expertise in data and evidence to produce outputs that support our partners and allies to better understand who has been left behind, in what ways, and why.
DI’s LNOB assessments have been developed to apply a systematic methodology that:
- Identifies and reviews relevant existing data.
- Analyses existing data to answer a locally relevant and targeted policy question.
During 2022 and 2023, four assessments were conducted in Kenya, Uganda, Benin and Nepal. Each assessment had a distinct focus that was identified and developed with local partners.
The LNOB assessment in Nepal sought to understand data and data infrastructure at the municipal level, considering how data can be used to inform local decision-making to reduce poverty and inequality. This approach was applied in two municipalities: Tulsipur and Simta.
This report presents the first part of the LNOB assessment in Tulsipur. It is based on DI’s data landscaping approach and assesses the range, quality and utility of existing data that can potentially inform issues relating to poverty and inequality in the municipality. It also assesses and makes recommendations about the underlying factors that could strengthen Tulsipur’s data ecosystem and enable improved and accessible evidence to be available in the future.
In November 2022, DI and Backward Society Education (BASE) held a co-creation workshop in Tulsipur. This was attended by representatives from Tulsipur’s municipal government, the Asia Foundation, the Sewa Foundation, the NGO Federation of Nepal, Didi Ghar and Apanaga Samhua. In the co-creation workshop, stakeholders identified priority research questions and discussed the methodological approach. Based on this, DI and BASE adapted DI’s general analytical framework for data landscaping in line with the set parameters. The team then conducted desk-based reviews of grey literature and face-to-face key informant interviews (KIIs) between December 2022 and January 2023. KIIs were conducted with 10 actors from 10 different departments of the local government. A final dissemination workshop was held in Tulsipur on 20 March 2023, with a total of 34 participants representing various organisations.
Part 1 of this report describes the quantity and quality of data included in the data inventory. Part 2 describes how this data is used in the municipality. Part 3 reviews the strength of the poverty and inequality data ecosystem as a whole, beyond the properties of individual data sources, and Part 4 provides recommendations for strengthening the local data ecosystem.
► Read our report on data landscaping in Simta, Nepal
► Discover more about DI's data landscaping approach
► Sign up to our newsletter
Part 1: Tulsipur’s poverty and inequality data inventory
The study team identified 11 data systems that provide information on poverty and inequality in Tulsipur; five administrative data systems, one survey and five mixed-method sources (i.e. unique sources that collate data from administrative systems, official surveys and censuses).
The identified systems produce data on social security payments (e.g. allowances for children and senior citizens); employment; asset ownership; education (e.g. enrolment rates and not-in-school numbers); health (e.g. vaccination and nutrition); violence against women; and disability (e.g. prevalence). The study team was unable to identify data on dimensions of poverty relating to voice and political participation.
Although the study team tried to identify unofficial data sources, they were unable to. [1]
Table 1: Inventory of Tulsipur’s five administrative data systems
Data system | What data is collected? |
---|---|
DRR Portal |
Type of incident (e.g. fire, animal incident, storm), location of
incident, number of people impacted by an incident and how (e.g. killed or injured), damage to infrastructure. |
Employment Management Information System
(EMIS) |
Information about applicants (ethnicity and gender, etc.). |
Health Management Information System (HMIS) |
Information on maternal and neo-natal health, nutrition, vaccination
and immunisation, and more. |
Integrated Education Management Information
System (IEMIS) |
Information on students, teachers and other staff. |
Vital Event Registration and Social
Protection management information system (VERSP MIS) |
Information on births, deaths, marriages, divorces and migration. |
Table 2: Inventory of Tulsipur’s five mixed-method sources
Data system | What data is collected? |
---|---|
Disability identity Card | Classifications of disabilities. |
Education data at district level |
Information about numbers of students and teachers by municipality and
school type. |
Judicial committee register |
Information on violence against women, people moving home and
divorce. |
Senior citizen identity card | Individual information: name, age and address, etc. |
Smart Daughter Programme |
Information about family members: name, address and place of birth,
etc. |
Table 3: Inventory of Tulsipur’s official survey
Data system | What data is collected? |
---|---|
Municipal Profile Survey |
Overall household survey including demographic profile, socioeconomic
profile, infrastructure, occupation, unemployment, etc. |
Disaggregation
In order to inform a leave-no-one-behind approach, it is necessary to identify individual and group-based characteristics that may influence poverty outcomes. To enable this, data must capture variables relating to multiple dimensions of poverty, such as health or access to electricity, but also include variables that can allow for disaggregation by characteristics that may be associated with inequality and exclusion within a population, such as gender, age or geography.
All of the data sources included in the data inventory produce data disaggregated by gender. Therefore, we can conclude that gender disaggregation has been successfully mainstreamed into data collection activities in Tulsipur.
Conversely, data disaggregated by geography (i.e. down to the ward or facility levels) is only collected by six of the data systems, and data disaggregated by age is only collected by five of the data systems (this does not include the education data available to us, as this was disaggregated by grade or year group).
In addition to this, only three of the data sources produce data disaggregated by ‘ethnicity’. [2] However, the lack of data disaggregated by ethnicity is not always due to this data not being collected. For example, administrative data is collected by ward offices when they issue disability identification cards. Initially, information is recorded on paper forms, and it is subsequently uploaded to an Excel file. Ethnicity data is collected on the paper forms, but it is routinely omitted from digital uploads. The paper forms then sit in storage and are not used. Stakeholders say the omissions are due to limited digital and human resource capacities.
The type of disaggregated data collected least often is (types of) disability data. This data is only collected by one of the identified data systems.
The omissions of these characteristics (e.g. geographic location, age, ethnicity and disability) from datasets prevents users from being able to use those datasets to generate insights about these dimensions via quantitative analysis (e.g. investigating how disability intersects with health outcomes), and significantly limits the range of available evidence. Collecting and digitally storing disaggregated data across all data systems in Tulsipur would better enable intersectional analysis.
Frequency
Ward offices upload civil registration data (e.g. birth and death registrations) to VERSP MIS on a daily basis, and Nepal Police also upload data to the DRR portal on a daily basis. This means users get to access this information in real time. [3]
However, data is collected and uploaded to other systems on a much more infrequent basis, meaning actors rely on information that may no longer be accurate or relevant. For example, data for the IEMIS is collected twice a year – at the beginning and end of the academic calendar – and is uploaded to the digital system by headteachers and the municipality’s education department around the same time it is collected. The schedule followed for the Prime Minister's employment program is similarly infrequent; data for it is only collected once a year (in February and March) and is uploaded to the digital system about a month later (in March and April).
Data is collected via the Municipal Profile Survey even less frequently: every five years. This infrequent scheduling is due to the costs of carrying it out. Therefore, despite the survey's capacity to produce good-quality statistics, it is not administered regularly enough to be relied on as the main source of evidence for local decision-makers.
Data sharing and open data
Data from the identified systems is generally not accessible in Tulsipur municipality; data from only three of the identified data systems is openly available, and in the case of two of these data systems, this is in the form of aggregated statistics, not microdata. Inaccessible data creates an obvious blockade preventing data use.
However, there is reason to believe this will change in the future. Tulsipur’s municipal government recently established an Integrated Data Management System (IDMS). The IDMS will pull data from other data systems semi-automatically, store it in a central database and display it on an open webpage. Presently, it is being piloted and some examples of anonymised data on civil registration, cooperatives, health, education, and agricultural services are available through it.
While the implementation of the IDMS is good for data sharing and open data, it does raise concerns about privacy. This is because there is a risk that the individuals or communities the published data is about will be identifiable. Nepal’s federal government has taken steps to make data protection more robust, namely through Article 29 of the Constitution (2015), the Individual Privacy Act (2075), the Muluki Criminal Code (2076), and the Individual Privacy Regulation (2077).
However, the majority of staff in Tulsipur who will upload data to the IDMS are not aware of these laws.
Ensuring staff are not only aware of these laws but also understand and abide by them is critically important. If this is done it will mean standards are kept, and it will reduce the risks associated with individuals relying on their own discretion to judge if something should or should not be published. This is especially important from a poverty and inequality perspective: if sensitive data about vulnerable individuals/communities is uploaded to the IDMS, it could put these people at a disproportionately increased risk of harm.
Metadata
Complete metadata allows actors to understand the data they are working with more promptly and thoroughly, and this encourages data use. Four of the 11 sources are accompanied by complete metadata; these are the VERSP-MIS, IEMIS, Health Management Information System and Employment Management Information System. A further four of the 11 data sources are accompanied by partially complete metadata. For example, the single Excel sheet where data for the senior citizen identity card, Smart Daughter Programme and disability identity card is collated, contains some metadata but is missing critical information such as the exact date the data was collected.
Part 2: The use of poverty and inequality data in Tulsipur
In general, the use of poverty and inequality data in Tulsipur is relatively low. For the most part, decisions are based on other priorities because actors have low levels of data literacy, do not fully appreciate the importance of evidence, perceive the quality of the available data as too poor to use, [4] and/or make decisions to meet other objectives (such as maintaining patronage networks).
Accessibility, particularly of microdata, is also a challenge that obstructs more data use. The culture of not sharing data is common across Nepal, and it even pervades the federal level of government: for example, the national statistical office does not currently have a policy for sharing or selling microdata, and, as such, it is not planning to share the latest census data with municipalities. However, the establishment of an Integrated Data Management System (IDMS) means that Tulsipur municipality is well placed to make improvements.
Despite these challenges, a number of interviewees told us they are interested in developing their use of evidence. For example, the Deputy Mayor is planning to identify key indicators for measuring poverty in the municipality, which align with local needs, and will prepare an index based on them. However, the municipal government has not yet drafted a plan on how this will be achieved. Moreover, other participants at the project workshop suggested that increased data use could be encouraged by departments attempting to analyse existing data, identify problems with it (such as data gaps), and find solutions (e.g. additional indicators) that can be applied during upcoming data-collection cycles.
Part 3: The foundations of Tulsipur’s poverty and inequality data ecosystem
Governance and management
Issues with the foundational components of Tulsipur’s local data ecosystem are unpacked in the following subsections of this report. Some analysis relates entirely to poverty and inequality, whereas the focus of other analysis is more general but equally significant.
ICT infrastructure and human resources
The information department is the municipality’s central body that should manage IT systems and data. Interviewees informed us that it has trained staff and sufficient ICT infrastructure. However, interviewees also reported that in most of the municipality’s other departments, there are too few staff who are adequately trained. This greatly restricts departments’ abilities to support data systems and conduct analysis for decision- and policy-making purposes.
“The employment department only has access to two staff that can support our work on data, and due to demands for their skills they have to shuffle between our department and others.”
Interviewee working in Tulsipur’s employment department, 2022
However, interviewees report that Lumbini’s provincial government has invested in training and skill development at the municipal level to boost officials’ digital literacy and their ability to use some specific information systems. For example, the province has already trained officials in the education department and is in the process of training officials from the health department. In addition to this, the Data for Development Program – a multi-year project funded by the UK’s Foreign, Commonwealth and Development Office – has provided training on digital literacy to municipal officials. These types of interventions need to be provided more regularly and to a wider group of stakeholders.
Cross-departmental coordination
Coordination on data by different stakeholders is a cornerstone of advanced data ecosystems. Effective coordination is difficult to achieve, but it can reduce the costs of data-related activities (e.g. collection and analysis), reduce data gaps and duplication, increase data access, and improve data quality.
Unfortunately, there is a severely restricted culture of coordination on data in Tulsipur municipality among departments, as highlighted by participants in the project workshop.
However, despite the absence of any formalised coordination on data across the municipality, some informal coordination between departments does happen in order to facilitate data sharing for specific purposes. For example, the health department shares data on malnutrition with the education department, and based on this data the education department prepares plans for midday meals in schools. But this type of coordination is cumbersome and relies on personal relations; it does not extend to collaborating on departmental data-collection exercises or analysis.
Workshop participants recommended establishing a unit or division at the municipal level to support data-informed policy and planning. They suggested that it should also work on cross-departmental data sharing. [5] Moreover, the Deputy Mayor proposed to work with departments and relevant organisations to improve joint departmental data management.
Legislation and policy
The Local Government Operations Act (2017; LGOA) outlines the responsibilities of local governments. It stipulates that municipal governments are responsible for poverty alleviation and the collection of the statistics required to fulfil their mandates. Therefore, local governments have a legal requirement to collect data related to poverty alleviation. The government in Tulsipur is aware of this. However, it has not designed a specific policy that would enhance its collection and management of poverty and inequality data. At present, this is being done on an ad-hoc basis by individual departments.
Tulsipur’s municipal government did prepare its own general ‘Statistics Act’ (2077). However, this contravened federal law and, therefore, was never fully instituted and is not practised. Despite this, the fact such an exercise was undertaken indicates that there is a general willingness to work on and improve municipal data.
Budget
An analysis of the budgets for health, education, social security, civil registration, land management, the environment, and disaster management, shows that a very small fraction of spending is directly allocated to data-related activities. The total budget for FY 2079–80 was NRs 1,864.78 million: only 0.14% of this was allocated to data collection and management.
Table 4: Budget allocated to data-related activities in select sectors
Department |
Activity
(2078–79) |
Budget
(2078–79) |
Activity
(2079–80) |
Budget |
---|---|---|---|---|
Health |
Purchasing computers and enabling connection (digitising health
institutions) |
NRs 600,000 (US$4,500) |
Purchasing computers and enabling connection (digitising health
institutions) |
NRs 200,000 (US$1,500) |
Land management |
Data and record collection on landless squatters, Dalit, and
unorganised dwellings |
NRs 1,800,000 (US$13,600) |
Data and record collection on landless squatters and Dalits,
unorganised dwellings |
NRs 2,000,000 (US$15,098) |
Municipality special project and plan | Digitisation of IT | NRs 500,000 (US$3,800) | ||
Social security and registration
department |
Orientation programme on civil registration | NRs 200,000 (US$1,500) |
Part 4: Recommendations
This section outlines top-level policy recommendations based on the findings detailed in parts 1–3.
Data sources:
- Ensure data disaggregated by ethnicity is digitised.
- Increase the frequency of data collection and the speed at which it is uploaded into digital systems.
- Continue the development of an Integrated Data Management System (IDMS).
- Ensure staff who upload data to the IDMS are aware of, understand and adhere to the protocol set out by data-protection legislation.
- Train administrative staff who input data into data systems in basic statistical concepts, plus the specific software they work with.
- Ensure complete metadata is collected and published alongside datasets.
Data use:
- Support the Deputy Mayor in their efforts to bolster data use in the municipality.
- Train key staff so they have the skills to conduct basic data analysis.
- Identify what data key users need and prioritise its publication through IDMS.
Data governance and management:
- Ensure there are enough staff with requisite skills to effectively manage and deliver sectoral departments’ data-related activities.
- Ensure all departments and other stakeholders are aware of IDMS and its potential to effectively share data.
- Enable a department (e.g. the information department) to take a greater lead in coordinating data-related activities across the municipality.
- Increase the proportion of budgets allocated towards data-related spending (e.g. training, hardware and coordination).
- Design and publish a strategy on how the municipality’s data ecosystem will be strengthened and how departments will coordinate/collaborate with each other on data-related activities.
Our recommendations aim to strengthen the local data ecosystem in Tulsipur, support evidence-informed policymaking and service provision, and contribute towards a reduction in poverty and inequality. To enhance data quality, disaggregated data must be digitised, the frequency of data collection needs to be increased, and digital systems must be used to expedite data sharing. Training staff in data analysis, statistical concepts, and the use of relevant programmes is also key to developing a more sophisticated data ecosystem. A municipal strategy for cross-departmental data sharing, as well as the greater allocation of resources to data infrastructure development and training, can also help to positively transform the collection, use and management of data in Tulsipur.
Annex
Table A1: Data systems and processes
Systems used | Who collects data? |
How often is it
collected? |
What data is collected? |
Where is the data input into
the digital system and by whom? |
Who uses the data? |
---|---|---|---|---|---|
Disability identity Card |
Ward Office staff and Department of Women, Children and Social
Development |
Ad hoc | Classifications of disabilities |
Department of Women, Children and Social Development record data in
register and computer (Excel sheet) |
Ward Office, Registration Department and Planning Department use the
data for decision-making |
DRR Portal | Nepal Police | Daily |
Information about incidents, such as fires and
storms, and their impacts (e.g. loss of life) |
By police officers | Local Emergency Operation Center and Local Disaster Management Team |
Education data at district level |
Schools: headteacher and concerned focal person from education
department at the municipality |
Twice a year |
Student information by school and municipality and teacher information
about private and government Schools |
School administrative staff and municipality education department | Schools, local government, district unit and province level |
Employment Management Information System
(EMIS) |
Ward Office staff | Once a year in February and March | Information about applicants (ethnicity and gender, etc.) | Employment Department staff |
The municipality, Ward Office, Registration Department and Planning
Department use the data for decision-making |
Health Management Information System (HMIS) | Female health volunteers and health workers | Data is collected daily and uploaded to the digital system monthly |
Information on maternal and neo-natal health, nutrition, vaccination
and immunisation, and more |
Health post and municipal health section |
Health post and municipal health centres, department of health
services at federal level |
Integrated Education Management Information
System (IEMIS) |
Headteachers and a focal person from the education department at the
municipality |
Twice a year: beginning and end of the academic year |
Information on students,
teachers and other staff |
In schools by headteachers |
Schools, central, provincial and local governments, and District
Education Coordination Units |
Judicial Committee Register | Department of Women, Children and Social Development |
It is collected when any incident is reported in the municipality by
the concerned person |
Information on violence against women, people moving home and divorce |
Department of Women, Children and Social Development record data in
register and digitally (Excel sheet) |
Deputy Mayor’s Office and Department of Women, Children and Social
Development |
Municipal Profile Survey | Municipality office | Every five years |
Overall household survey including demographic profile, socioeconomic
profile, infrastructure, occupation, unemployment, etc. |
Information Department | Planning and budgeting, making policies and reporting |
Senior citizen identity card |
Ward Office staff and Department of Women, Children and Social
Development |
Data is collected when individuals register for services in the ward
office |
Individual information: name, age and address, etc. |
Department of Women, Children and Social Development record data in
register and digitally (Excel sheet) |
Ward Office, Registration Department and Planning Department use the
data for decision-making |
Smart Daughter Programme |
Ward Office staff and Department of Women, Children and Social
Development |
Data is collected when families submit details |
Information about family members: name, address and place of birth,
etc. |
Department of Women, Children and Social Development record data in
register and digitally (Excel sheet) |
Ward Office, Registration Department and Planning Department use the
data for decision-making |
VERSP MIS | Staff at ward office | Daily | Information on births, deaths, marriages, divorces and migration | Staff at ward office |
Ward office, municipal government, department of national ID and civil
registration, and Central Bureau of Statistics |
Table A2: Municipality data-related responsibilities as per the Local Government Operations Act (2017)
Areas |
Functions and duties related to
data |
---|---|
Data collection about unemployment |
Data collection, processing and information system establishment for
the employed and unemployed labour force Domestic and foreign labour records management |
Disaster management | Data management of local disasters |
Health | Health insurance and social security management programme |
Landless squatter management | Identification and record management |
Livestock | Data management and information system on local livestock |
Local data and records compilation |
Policy, law, standards and regulation related to local data
Basic data collection and management Registration of births, deaths, marriages, divorces and migration Acts related to local data management |
Local records management |
Formulation and Implementation of policies, plans and standards for
local records management Use of information technology in records management |
Personal incidents registration | Vital registration and records management |
Social security and poverty
alleviation |
Local policy, standards and legislation for social security and
poverty alleviation Social security-related data management and information management Identification of households living in poverty and information management |
Downloads
Notes
-
1
Obtaining unofficial data in small localities is notoriously difficult without pre-existing relationships with local organisations. Therefore, the study team not being able to identify any unofficial poverty and inequality data in Tulsipur does not necessarily mean there is not any.Return to source text
-
2
‘Ethnicity’ and ‘caste’ are used interchangeably across the different data systems. We decided to use ethnicity because slightly more systems use this term.Return to source text
-
3
VERSP-MIS data suffer from some time lag caused by families/guardians reporting events to ward offices after the specified time limit (i.e. 35 days).Return to source text
-
4
For example, the participants of the project dissemination workshop in Tulsipur expressed particular concerns about the validity of data in the Municipal Profile Report (2017/18). They said the process of data collection was not consultative, the data was not validated before it was published as a report on the municipality’s website, and they suggested the need for more disaggregated data, particularly by ethnicity, to identify which groups are most affected by poverty and inequality.Return to source text
-
5
Guidelines stipulate that officers in the information department should work with other departments to obtain the data they collect and disseminate it. This means the information department should have oversight of data-related activities in the municipality and could play a coordination role. However, interviewees report that the department's interactions with other departments do not currently extend to data, let alone facilitating cross-departmental data coordination.Return to source text
Related content
LNOB assessment in Nepal: Data landscaping in Simta municipality
This report, co-produced with BASE, draws on DI's data landscaping work in Simta, Nepal. It explains how to improve the data ecosystem to leave no one behind.
The data side of leaving no one behind
In this paper we look at lessons learned from data landscaping at the national level, and examine how it can be used to inform decisions about poverty eradication and ensure no one is left behind.